UNITED STATES—Clinical data management (CDM) collects, cleans, and manages clinical data to ensure accuracy, completeness, and compliance with regulatory standards. CDM is vital to clinical research, giving researchers valuable insights into the safety and efficacy of new drugs and treatments.
However, researchers face challenges in clinical data management. The amount of clinical trial data is increasing exponentially, making storing, managing, and analyzing data difficult. Data security is also a risk, wherein clinical data is a prime target of cybercriminals, prone to unauthorized access, disclosure, and alteration.
Clinical trial facilities and contract research organizations (CROs) must implement the best CDM practices and guidelines to overcome these challenges. Read on below to find out more.
Establish A Data Governance Framework
A data-governance framework governs clinical data collection, storage, and use. A well-designed framework can help ensure clinical data is accurate, complete, and secure.
The Clinical Data Interchange Standards Consortium (CDISC) and Study Data Tabulation Model (SDTM) are important factors in establishing a data governance framework. The CDISC sets data standards to ensure that data collected during clinical trials is consistent and accurate, and can be easily shared across research organizations.
The SDTM is one of the CDISC standards that provides a format for organizing and presenting data from clinical trials. SDTM defines how data should be collected and organized into tables, making it easier for researchers to analyze and compare data from different studies.
Incorporating CDISC standards, such as SDTM controlled terminology, into a data-governance framework can help ensure that data is collected consistently and accurately across different studies and research organizations. This can improve the quality of research and ultimately lead to better healthcare outcomes.
Implement Data-Validation Processes
Data-validation processes aim to identify and correct errors in clinical data manually or using automated tools.
With data validation, a clinical research facility can boost efficiency, reducing data collection, analysis, and reporting time and cost. Data validation can also help reduce the risk of regulatory noncompliance.
Clinical data automation tools are software applications that help to automate the collection, management, and analysis of clinical data. An example is electronic data capture (EDC), discussed below.
Use Electronic Data Capture Systems
Modern clinical trials use electronic data capture systems. EDC systems are software applications that automate the collection and management of clinical data. EDC systems can help improve the accuracy and completeness of clinical data and reduce the time and cost of data entry.
One excellent feature of an EDC is customizable case report forms (CRFs), which can be formatted to meet the specific needs of a clinical study. Researchers use CRFs to collect clinical data from study participants.
The first step when choosing an EDC system is to understand the basic functionalities of the system and the value it can add to your organization. For example, a multisite study needs an EDC system supporting remote data entry. It is best to get quotes from multiple vendors for thorough deliberation before deciding.
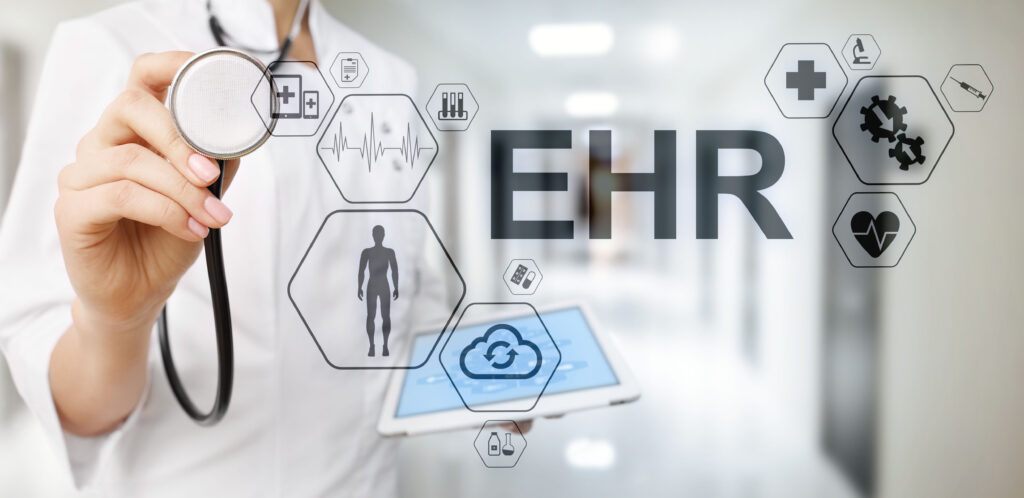
Use A Central Clinical Data Repository
A central repository for clinical data is a database that stores all of the clinical data for a particular study or organization. It centralizes data from multiple sources, such as electronic health records (EHRs), clinical trials, and research databases, improving the accessibility and usability of clinical data.
In addition, a central repository standardizes data by defining common data fields and formats. Researchers can easily share clinical trial data with colleagues, partner organizations, and regulatory agencies. Hence, a central clinical data repository can help improve the quality of data analysis, especially in studying new drugs.
Implement Security Measures
Cybersecurity breaches in clinical trial data can devastate patients, researchers, and the entire healthcare industry. They can lead to the unauthorized disclosure of patient data, identity theft, and fraud.
AstraZeneca blamed a user error (a password lapse) for exposing sensitive patient data on the code-sharing site GitHub. The credentials for an AstraZeneca internal server were left on the site in 2021, where they were exposed for over a year. It is not clear if any of the data was accessed or stolen. This error is common in the pharmaceutical industry and can lead to widespread cybersecurity vulnerabilities.
Clinical data is confidential and must be protected from unauthorized access, disclosure, or alteration. Robust security measures must be implemented to ensure the confidentiality and integrity of the clinical trial data throughout the data-management process. This includes implementing access controls, encryption, and regular backups to protect against data loss or corruption.
Provide Clinical Staff Training
Clinical staff who collect, enter, and manage clinical data should be trained in the best practices for CDM. Training should cover topics about data entry, data validation, and data quality, to name a few. Before deployment, CROs must ensure that all clinical staff members undergo intensive training for clinical data management.
Conclusion
Implementing the best practices and technologies can help ensure a successful clinical trial. By following the best practices above, clinical trial facilities and contract research organizations can improve their clinical data’s accuracy, completeness, and compliance. This can lead to improved decision-making in clinical research and improved patient care.